AI technology is developing at a dizzying pace, so what do community banks need to know about how to get started with AI, how it can be used to boost efficiency across departments—and how to manage the risks?
Independent Banker spoke to experts from ICBA and beyond to learn about the potential of this incredible technology for the banking industry.
Who's in conversation
Saroop Bharwani, CEO, Senso, Toronto, Canada
Slaven Bilac, Co-founder and CEO, Agent IQ, San Francisco
Robert Johnston, CEO of Adlumin Inc., Washington, D.C.
Wayne Miller, Senior vice president of innovation programs, ICBA Solutions Group
Charles Potts, Executive vice president, chief innovation officer, ICBA
What AI technology is available to bankers today, what’s coming up soon and what they can expect in the next few years?
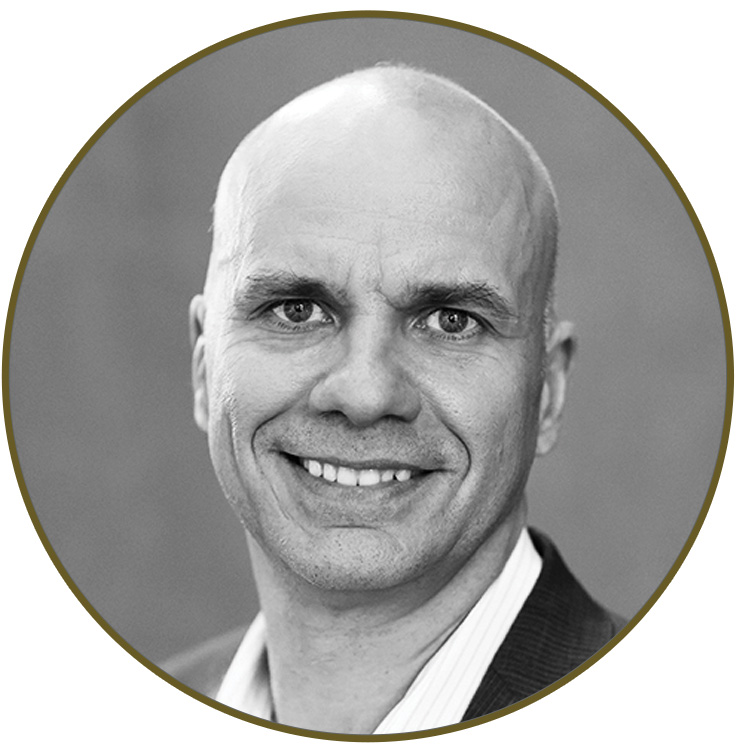
Slaven Bilac: While the buzz around AI has greatly increased with the release of ChatGPT, AI has been around for much longer, with many applications in the banking space, including customer service, risk analysis, underwriting, security, fraud prevention, regulatory compliance and others.
What is important to keep in mind is that AI is a general term that encompasses many different tools, techniques and actual applications that will typically use just a very focused subset to solve—or reduce—a pain point. That said, tools and techniques are becoming more powerful, and it is reasonable to expect the impact of these using AI will increase exponentially in the next few years as wider, more practical applications become possible.

Charles Potts: It’s important to recognize that for decades, community banks had been using various legacy forms of AI, primarily in the card fraud area and in credit scoring models—though many may not have been aware that they were AI-powered.
There are still legacy AI models out there, but as we now look at the evolution of technology and the cost effectiveness it has brought, the idea of using generative AI in other parts of the bank is becoming more and more of an acceptable practice.
Therefore, we really are in the era of experimentation on the part of community banks—trying to figure out exactly what it is and how they can best use it to the benefit of their customers and to the bank itself.
Banks are deploying AI within their compliance activities, engaging in some Know Your Customer (KYC) and Know Your Business (KYB) analysis as part of their customer interactions. There is great promise and potential for using some of the AI tools out there to comb through large amounts of data and assessing patterns, anomalies and validation. There is also the potential to make compliance more efficient and/or speed up the process for banks and their customers.
Quick Stat
$407B
The value the AI market is expected to reach by 2027
Source: MarketsandMarkets
AI also has great promise in the customer service function. We’re seeing deployments behind chatbots—AI technology that can comb through mounds of bank information and provide very efficient interactions with customers. Banks need to make sure the data they allow those tech platforms to use is very specific to their bank, and that’s where closed-loop AI engines are finding great promise.
Back-office functions that have a lot of consistently repetitive processes are other good areas where we’re seeing some early-stage companies playing in the AI field. Even with things like audit functions—post-closing audit and examination—AI can comb through massive amounts of information efficiently, and there’s great potential for AI in the back office as well.
“AI is quickly becoming a standard in how we assess and manage fraudulent activity as well as helping bankers more efficiently market their services to their customers because they can better understand their specific needs.”—Wayne Miller, ICBA Solutions Group
There are also some innovative tech companies offering ways for banks to deploy AI into the routine and repetitive reporting for regulatory call reports and even for their own board packages that they put together every month and quarter. And more bankers are using AI to help them draft presentations or a complex email need, just like I do. AI can go grab information that I need, and I can edit and shape the document to my own voice and perspective.
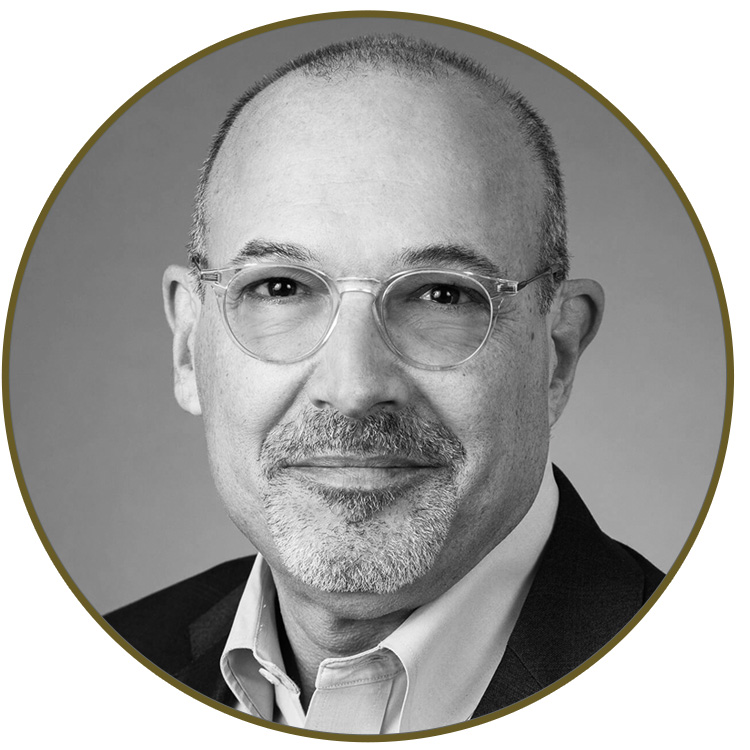
Wayne Miller: I think that today, AI can be impactful in improving customer service and support— helping employees in the bank be more productive and efficient, as well as providing a platform for internal learning and knowledge.
AI is quickly becoming a standard in how we assess and manage fraudulent activity as well as helping bankers more efficiently market their services to their customers because they can better understand their specific needs.
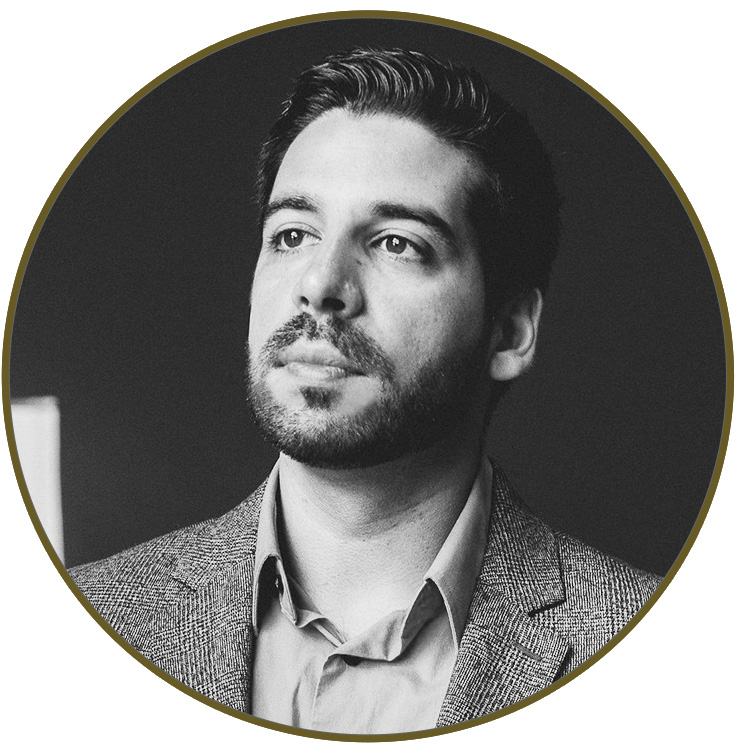
Saroop Bharwani: The concept of AI has been in development for eight decades, but a pivotal moment occurred on Nov. 30, 2022, with the launch of ChatGPT. [It demonstrated] the value of generative AI and large language models to the world.
The technology available to bankers today is a form of AI that, when given [an input], leverages a statistical model to predict an expected response, creating new, context-relevant content. More specifically, large language models are very effective at predicting the next word.
When you ask a question to ChatGPT, it generates a response by predicting the most likely sequence of words to follow your input by drawing on a vast corpus of text it has been trained on across the internet.
Within the next decade, ubiquitous intelligence will be accessible to everyone with a smart device, effectively giving everyone their own intelligent chief of staff for a variety of tasks, from organizing schedules, answering emails, booking flights, refinancing mortgages and performing a host of other tasks across banking workflows.
What risks should bankers be aware of around AI?
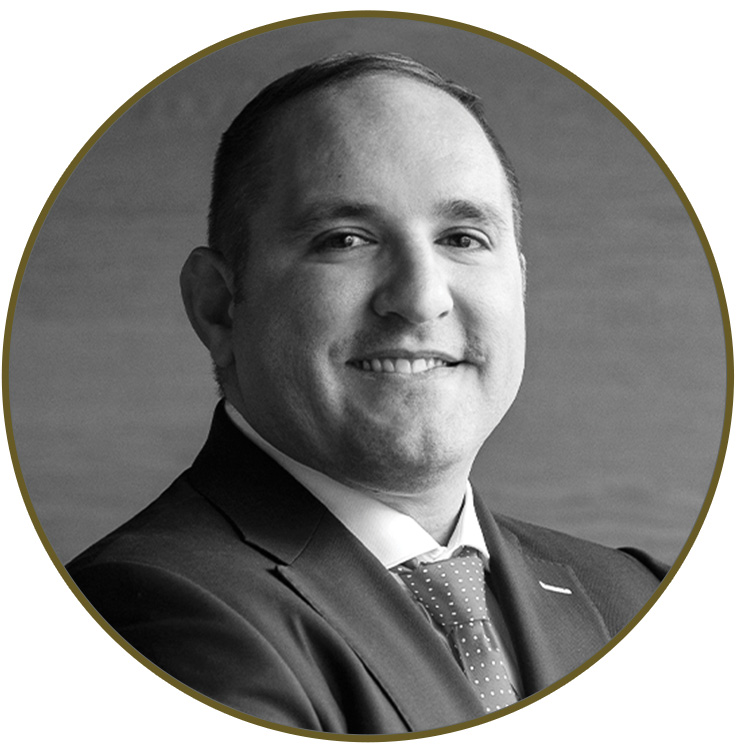
Robert Johnston: Data quality and availability are top of mind when building viable and useful AI applications. Machines can only train on reliable data for the output to be actionable. Great attention is therefore required in building a robust infrastructure for sourcing, processing, storing and querying the data. Not securing a chain of custody for input data means AI applications are at risk of generating misleading output.
Community banks should be aware of the limitations and “biases” of any machine-learned prediction and need to maintain visibility into AI model characteristics, like “prediction accuracy tends to falter beyond a certain range of input values” or “some customer groups were underrepresented in the training data.”
Operationally, a good way to proceed is to build and deploy a series of increasingly complex AI applications, rather than being wedded to an ambitious design at the get-go. Iteratively adding functionality and gradually incorporating more data fields can make measuring performance easier and avoid costly mistakes.
Bilac: Bankers should understand that AI is not a do-it-all, perfect solution for every problem. It is most effective when applied to narrow and practical problems where it is easy to deploy and measure the benefits of using the technology. Because of this, vendors who make wide-ranging promises about their AI systems should be considered with a due level of scrutiny and diligence.
“Banks need to be circumspect and thoughtful about potential data bias in the AI models themselves and always check their work.”—Charles Potts, ICBA
Another potential pitfall I have seen some banks run into is jumping in too early and trying to develop their own systems from scratch. Open sourcing and the publication of algorithms has made the basic ingredients for AI tools readily available, but it still takes a significant amount of expertise to take these foundational elements to a state ready for implementation, evaluation, deployment and maintenance. This approach can be quite resource-intensive and in most cases turns out to be much more complicated and expensive than initially imagined.
Potts: Banks need to be circumspect and thoughtful about potential data bias in the AI models themselves and always check their work. Banks also need to be mindful of the transparency of the models they use; this is where the regulatory bodies are keenly interested. You can’t just have these black box processes in place that you can’t explain to examiners and auditors. The models that get used have to have some real transparent “explainability” to them.
The third issue is overreliance, especially for community banks because the relationship banking aspect of community banking continues to work for a reason.
Bharwani: Over the past year, I’ve had the pleasure of engaging with hundreds of financial services executives on the transformative potential of generative AI, while also discussing risk mitigation and use-case selection strategies. The question that I get asked most frequently is how to harness the power of these models on their proprietary institutional knowledge without exposing sensitive data.
The reactive solution for many is to block tools like ChatGPT within their organization. However, this does not address risks that arise from personal device usage, effectively reducing organizational visibility into data exposure.
In my discussions with banking executives, I focus on how they can integrate AI into banking workflows in a manner that’s both low risk and high impact. The aim is to reduce data exposure risks while enabling better control and oversight of AI model usage within their organizations.
Ultimately, how will AI allow community banks to improvetheir own processes and better assist their customers?
Johnston: The promise of AI is to empower business analysts and leaders with enriched information to make profitable decisions while delivering a delightful customer experience. Selecting a product marketing strategy, predicting revenue differentials from alternative interest-rate offers, improving in-branch or online customer experience, and providing research and decision-making tools to customers—all these problems are best addressed via a data-driven approach.
“It is important for banks to understand what makes them unique and worthy of their clientele and pick AI applications that will help them preserve the essence of their brand while making the customer experience better and processes more efficient.”—Slaven Bilac, Agent IQ
This applies to information security, too, of course. The sheer volume of security data can overwhelm monitoring and incident-response efforts unless enabled with machine-learned anomaly detection.
An overarching principle for building an AI suite is to be customer‑centric. This means first determining what’s important from a customer advocacy standpoint and then working backward to prioritize the development of AI capability.
Bilac: Rather than focusing on the technology, I would encourage bankers to focus on the specific problems they are trying to solve and think of using AI as a tool for, initially, a narrow role and then gradually expand as results and ROI manifested.
In addition, it is important for banks to understand what makes them unique and worthy of their clientele and pick AI applications that will help them preserve the essence of their brand while making the customer experience better and processes more efficient.
More from ICBA
In partnership with Senso, ICBA’s Demystifying AI webinar series educates community bankers on the far-reaching implications of generative AI in the financial landscape. Learn More »
For example, banks that value relationship banking as a core value should probably not deploy a chatbot experience with no ability to ever access a human. Instead, they should be congruent to their brand and deliver a hybrid model that allows them to couple the best of AI chatbot speed and availability with the empathy and relationship aspect of their own bankers to tackle complex and emotional tasks.
Miller: Banks, like all businesses, are also going to dedicate resources to this so they can increase their knowledge of AI. Lack of expertise is an issue, but that will change over time. They need to invest in gaining this knowledge for today and the future. Most are taking a phased approach to manage this both from a personnel perspective as well as a budgetary perspective.
Based on some data that I have seen, less than a third of banks are embracing this opportunity, with most waiting to see how this unfolds.
Bharwani: With rapid improvements in user experience, the imperative is clear: Financial services executives must strategically incorporate this technology into their daily workflows, and the impact will be noticeable.
The most effective methodology involves a “crawl, walk, run” approach, starting with low-risk, high-impact use cases that not only invigorate internal teams but also set the stage for more advanced applications.
Imagine a future where every customer interacts with a specialized AI assistant for seamless account management, money transfers or mortgage refinancing, all through a natural language conversation.
AI case study:
Rockland Trust Co. + Agent IQ
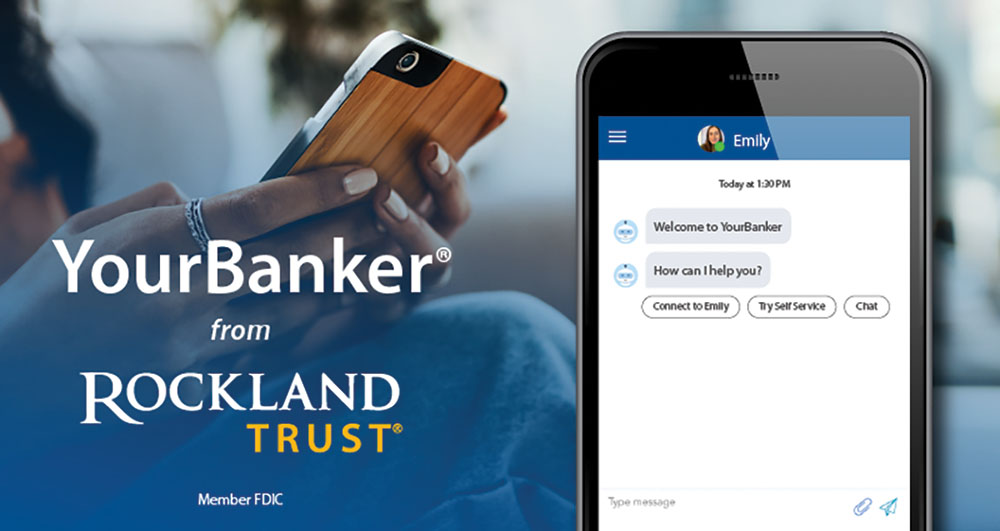
Rockland Trust Co. in Rockland, Mass., deploys AI-powered tools across a number of bank functions, says Patrick Myron, senior vice president, retail network strategy and sales analytics for the $19.4 billion-asset community bank.
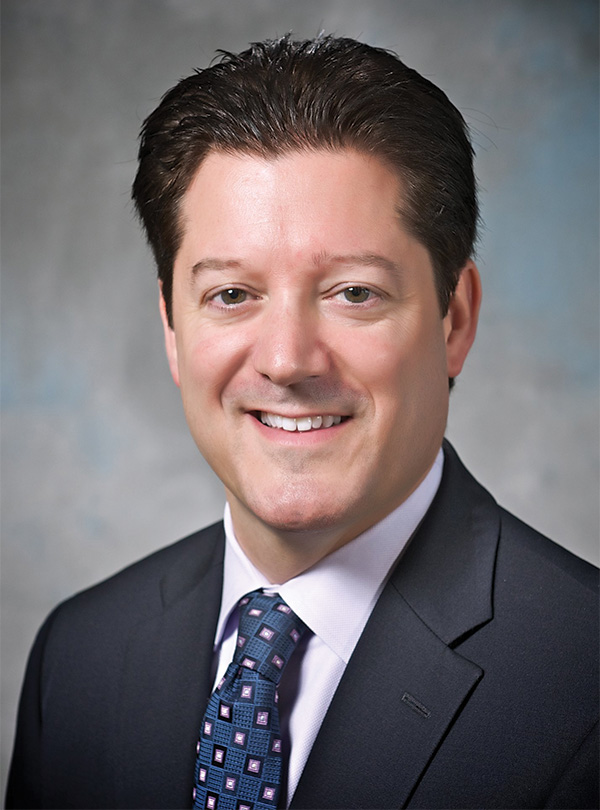
Rockland Trust partnered with fintech Agent IQ to offer its customers YourBanker, a digital tool that allows customers to chat and share documents securely with their own dedicated banker from their mobile device or computer.
“With YourBanker,” Myron says, “AI responds to customers and gives them options on how to get an answer for their specific question by allowing them to connect or leave a message for their own dedicated banker, choose self-service for answers to basic questions or chat with a banker immediately.”
In addition, AI can generate automated responses and suggest answers for the YourBanker agents to use as they have conversations with their customers. Since its launch in 2021, customers have logged into YourBanker more than 171,000 times.
“Customers can establish digital relationships anytime, anywhere with their bankers, who will help provide them with everyday banking support,” Myron says.
How AI mines data
As in most industries, AI technology is indispensable in banking for distilling actionable intelligence from the massive amounts of data being ingested from customers and generated by employees.
Community banks can choose from a vast range of available data mining and AI methods, depending on desired outcomes and data availability, says Robert Johnston, CEO of Adlumin Inc. in Washington, D.C.
“For example, if the goal is to evaluate each customer for digital marketing suitability for a new product, ‘supervised’ methods such as logistic regression or decision-tree classifier could be trained on customer data,” Johnston says. “These use cases require customer data on prior actions, such as historical responses to marketing emails.”
For a customer segmentation issue, “unsupervised” methods such as DBSCAN clustering or PCA dimensionality reduction are called for, where prior observations aren’t imposed on specific customer actions, but rather aim at customers according to machine-learned similarity measurements, he says.
More advanced methods, such as artificial neural networks (ANNs), are deployed when the use case depends on learning complex interactions among numerous factors, such as customer service call volume and outcome evaluation, or even the customer classification and clustering problems mentioned earlier, Johnston says. The data volume, frequency and computer capacity requirements are typically heavier for ANNs than for other machine learning techniques.
The most visible near-term evolution in the field is the spread of large language models or generative AI, such as ChatGPT, he says. The underlying methods behind these emergent AI technologies are also based on ANNs mentioned above—only with hugely complicated neural network architectures and computationally very expensive learning algorithms.
“Adaptation and adoption of these methods for customer classification, segmentation and interaction-facilitation problems will be a trend to closely follow in the years ahead,” Johnston says.
The central challenge for AI in cyber applications is to find “needle in haystack” anomalies from billions of data points that mostly appear indistinguishable, he says.
The applications in this domain are usefully grouped under user and entity behavior analytics (UEBA) and involve mathematical baselining of users and devices on a computer network followed by machine-identification of suspicious deviations from baseline, Johnston says.